Metrohm viva 1.0 (process analysis) User Manual
Page 629
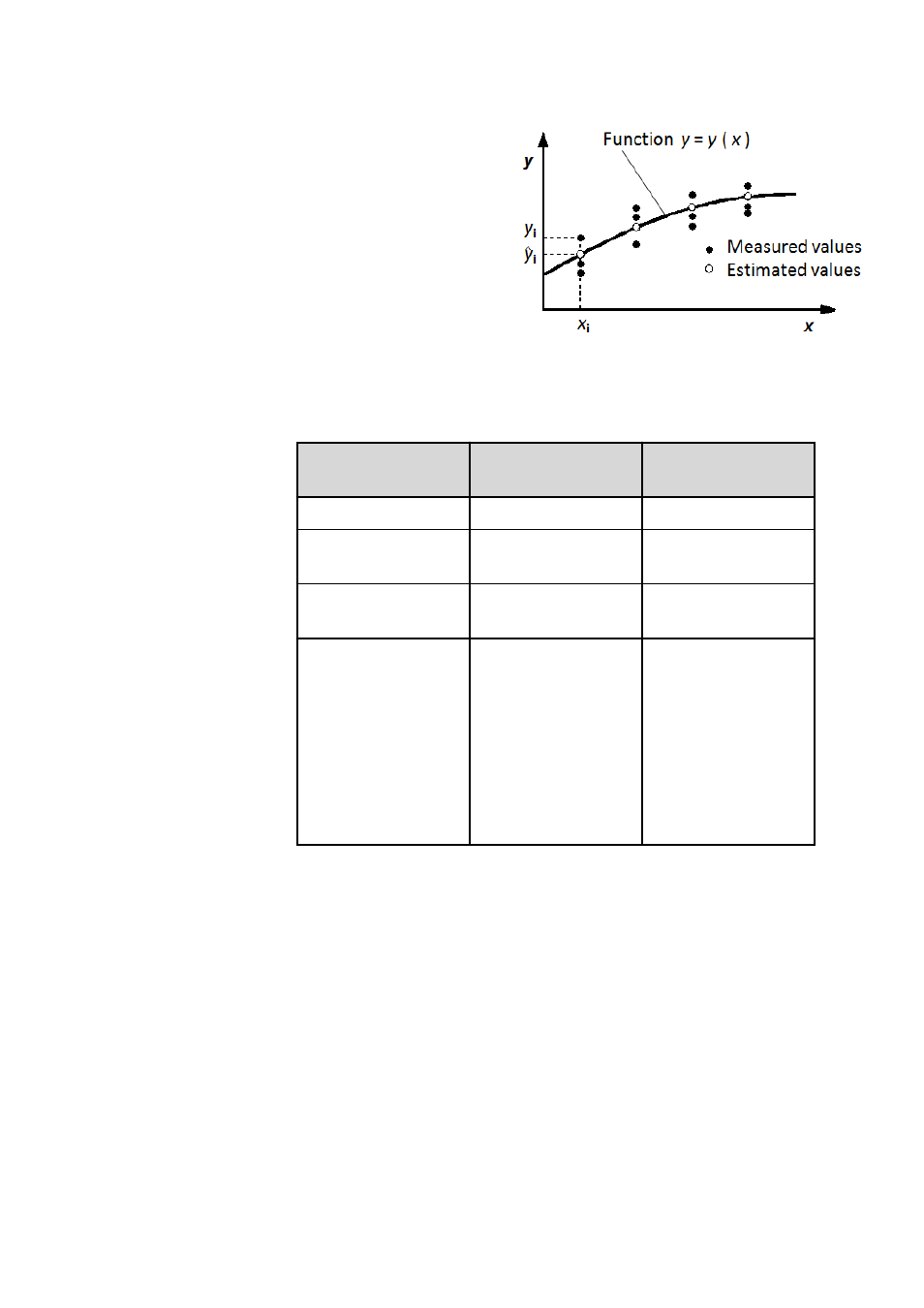
■■■■■■■■■■■■■■■■■■■■■■
5 Method
viva 1.0 (for Process analysis)
■■■■■■■■
617
■
The variable x is error-
free.
■
The variable y is
dependent on x and
can be described by the
function y = y(x).
■
The error with the mea-
surement of y is distrib-
uted normally and is
sufficiently small to be
able to apply linear
error calculation.
Depending on the calibration method selected, the following model func-
tions are available for the calculation of the calibration curve y = y(x):
Selected curve type
Calibration func-
tion
Description
Linear regression
y = a + bx
Line
Quadratic regres-
sion
y = a + bx + cx
2
Non-linear curve of
the 2nd degree
Nonlinear regres-
sion
y = a + bx + dx
4
Non-linear curve of
the 4th degree
Linear interpola-
tion
y = a + bx
Line for which all rep-
lications of the two
standard solutions
which are closest in
size to the measured
value of the sample
are taken into
account by the cali-
bration curve.
To calculate the parameters a, b, c and d, one proceeds in accordance
with the Least Squares Fit method, for which the sum of the squared devi-
ations of the measured values y
i
are minimized by the estimates
ŷ
i
. The
scatter
σ
y,i
of the measured values is usually not constant, however, but
rather dependent on its value. It is for that reason that the deviations can
be weighted with a factor of g
i
. Extremely scattered values should be
given less weight, more precisely measured values should be weighted
more heavily. It is known from statistics that, under the conditions listed,
weighting 1/variance = 1/standard deviation
2
= 1/(
σ
y,i
)
2
yields the best
results. In practice, however, one usually has too few repeated measure-
ments to be able to make estimates from the measured values
σ. A gen-
eral fact is of help here: