Linkage rules – UVP Life Science User Manual
Page 151
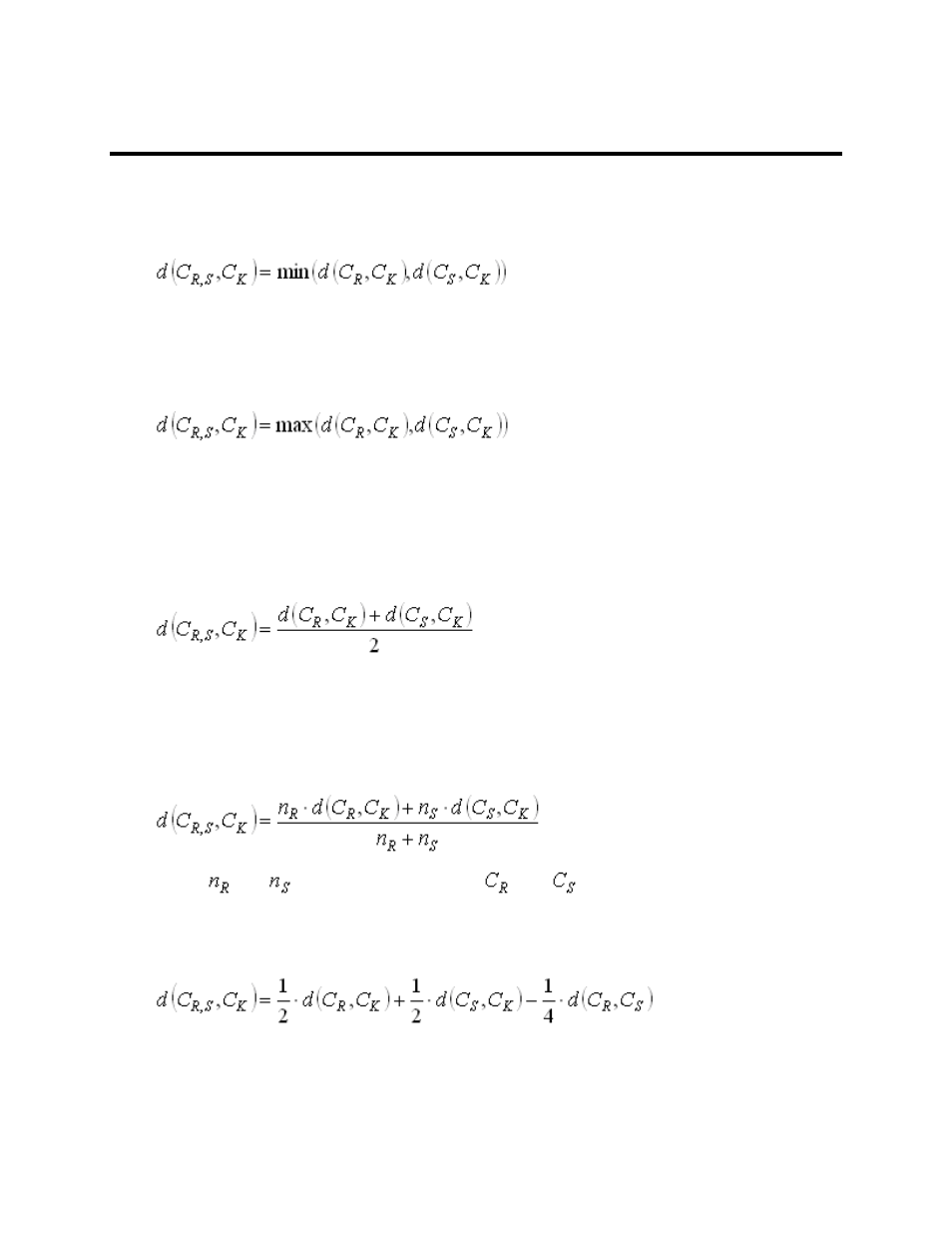
Perform 1D Analysis
137
Linkage Rules
A linkage rule offers a method to calculate a measure of the distance between two clusters.
•
Single Linkage (nearest neighbor): The distance between two clusters is given by the distance
between the two closest items (lanes) in the different clusters.
Using this method often causes the chaining phenomenon, which is a direct consequence of the
single linkage method tending to force clusters together due to single entities being close to each
other regardless of the positions of other entities in that cluster.
•
Complete Linkage (furthest neighbor): The distance between two clusters is given by the greatest
distance between two items in the different clusters.
This method should not be used if there is a lot of noise expected to be present in the dataset,
because outliers are given more weight in the cluster decision. It also produces very compact
clusters. This method is useful if one is expecting entities of the same cluster to be far apart in multi-
dimensional space (provided there is no noise).
•
Unweighted pair-group method average (UPGMA): The distance between two clusters is
calculated as the arithmetic mean of the distances between all possible pairs of entities of the two
clusters in question.
This method is a halfway choice between single and complete linkage. The chaining problem is not
observed for this method and outliers are not given any special favor in the cluster decision, which
makes this method the most popular.
•
Weighted pair-group method average (WPGMA): This is identical to UPGMA except that the
number of items in a cluster is taken into account &endash; this may be useful when there is a
large variation in the number of items in the clusters.
, where
and
are the respective sizes of
and
•
Unweighted pair-group method centroid (UPGMC): The distance between two clusters is the
distance between the centroids of each cluster (the centroid of a cluster is the average point in
the multidimensional space of the cluster).
The resulting trees are not right-aligned and branches can have negative values.