Image clustering – Kofax Getting Started with Ascent Xtrata Pro User Manual
Page 99
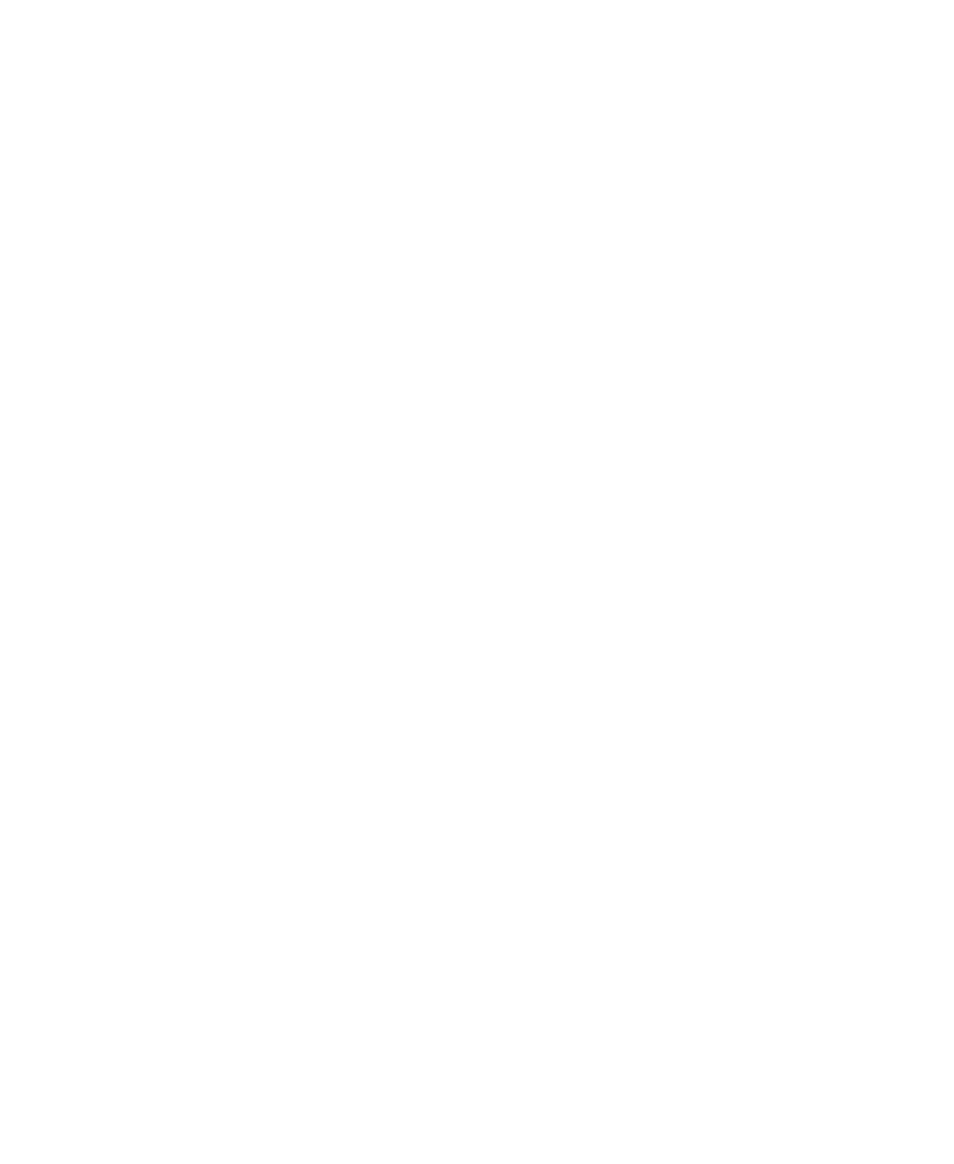
Chapter 3
80
Ascent Xtrata Pro User's Guide
Training
Max samples per class
The Layout Classifier supports an unlimited number of samples per class. If the
sample images are very different, the Layout Classifier internally learns different
patterns for each sample. For performance reasons, you might want to limit the
number of sample documents that are used for feature extraction. A value of 0
means no limitation.
Class homogeneity
This feature controls how sensitive the classifier is to variations in the layout of
the images in the training set. If the sample images are very different, the Layout
Classifier automatically creates internal patterns for each new type. These types
are not visible to the user.
The more types the better the classification accuracy, but the slower the
classification speed. The value set by this control is a threshold, which
determines when new internal types are created. In most cases the default value
of 80.0 works the best.
Noise Filter
This feature controls how to match regions with low contrast (for example,
images with a fine background pattern). A value closer to the “max. precision”
side would not classify images with low contrast. This means that even
documents from the training set would not have 100% confidence. The
probability of getting misclassified documents would then be much smaller,
resulting in a higher accuracy but more rejects. If you make the value closer to
the “max. recall” side, higher confidence values are returned for documents with
low contrast. However, this might mean that high confidence values are
determined for other classes with low contrast in the same region of the
document, which might lead to a higher error rate. In most cases the default
value of 15.0 works best.
Image Clustering
To facilitate set up of the Layout Classifier, a special function is provided that
performs automatic clustering (grouping) of unknown document images. The images
are clustered by geometrical similarity and can be easily added to the training set.