HP 49g+ User Manual
Page 619
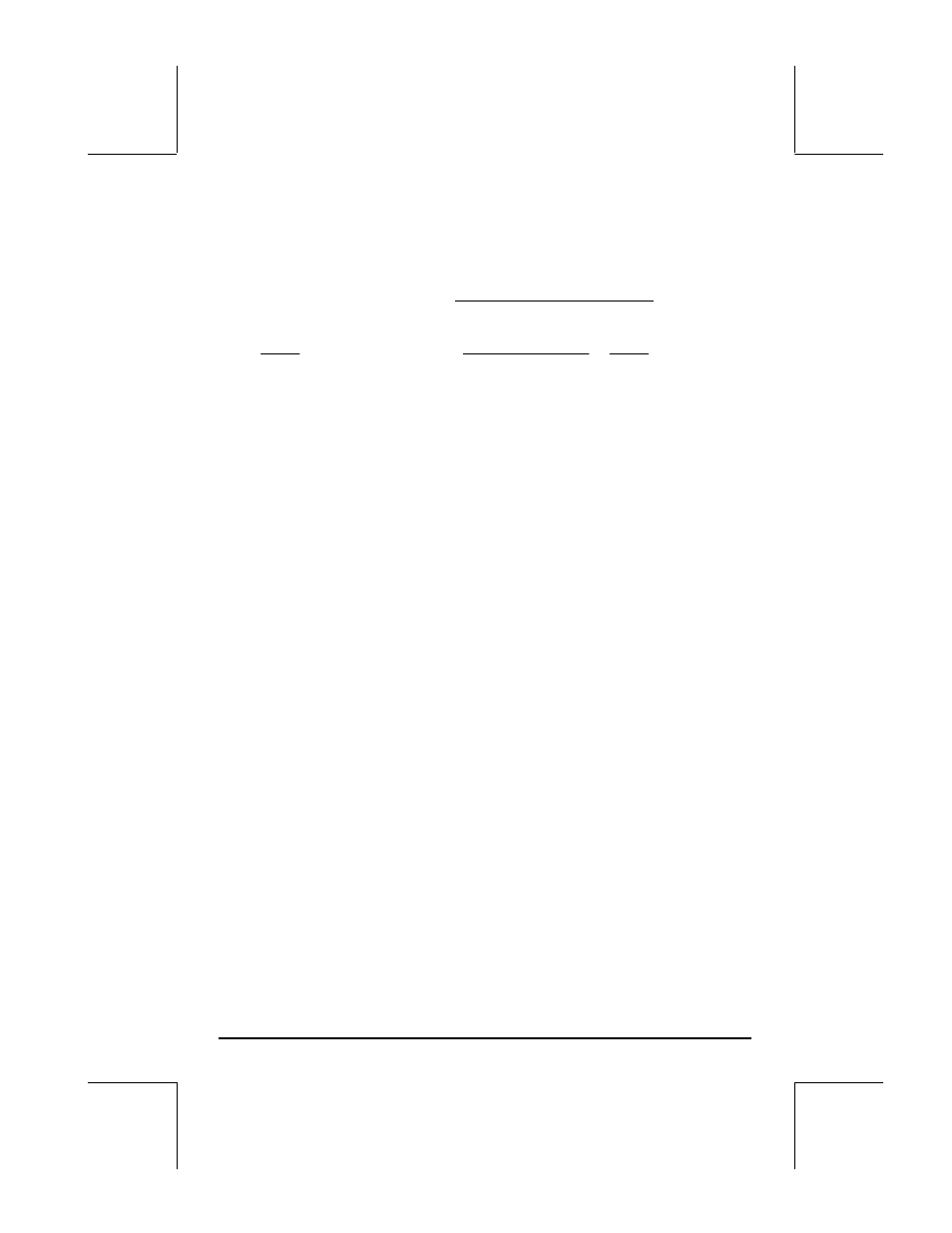
Page 18-52
Let y
i
= actual data value,
^
y
i
= a + b
⋅x
i
= least-square prediction of the data.
Then, the prediction error is: e
i
= y
i
-
^
y
i
= y
i
- (a + b
⋅x
i
).
An estimate of
σ
2
is the, so-called, standard error of the estimate,
)
1
(
2
1
2
/
)
(
)]
(
[
2
1
2
2
2
2
1
2
xy
y
xx
xy
yy
i
n
i
i
e
r
s
n
n
n
S
S
S
bx
a
y
n
s
−
⋅
⋅
−
−
=
−
−
=
+
−
−
=
∑
=
Confidence intervals and hypothesis testing in linear regression
Here are some concepts and equations related to statistical inference for
linear regression:
• Confidence limits for regression coefficients:
For the slope (
Β): b − (t
n-2,
α
/2
)
⋅s
e
/
√S
xx
<
Β < b + (t
n-2,
α
/2
)
⋅s
e
/
√S
xx
,
For the intercept (
Α):
a
− (t
n-2,
α
/2
)
⋅s
e
⋅[(1/n)+x
2
/S
xx
]
1/2
<
Α < a + (t
n-2,
α
/2
)
⋅s
e
⋅[(1/n)+x
2
/S
xx
]
1/2
,
where t follows the Student’s t distribution with
ν = n – 2, degrees of
freedom, and n represents the number of points in the sample.
• Hypothesis testing on the slope, Β:
Null hypothesis, H
0
:
Β = Β
0
, tested against the alternative hypothesis, H
1
:
Β ≠ Β
0
. The test statistic is t
0
= (b -
Β
0
)/(s
e
/
√S
xx
), where t follows the
Student’s t distribution with
ν = n – 2, degrees of freedom, and n
represents the number of points in the sample. The test is carried out as
that of a mean value hypothesis testing, i.e., given the level of
significance,
α, determine the critical value of t, t
α
/2
, then, reject H
0
if t
0
>
t
α
/2
or if t
0
< - t
α
/2
.
If you test for the value
Β
0
= 0, and it turns out that the test suggests that
you do not reject the null hypothesis, H
0
:
Β = 0, then, the validity of a
linear regression is in doubt. In other words, the sample data does not
support the assertion that
Β ≠ 0. Therefore, this is a test of the
significance of the regression model.