Confidence interval for a proportion – HP 49g+ User Manual
Page 591
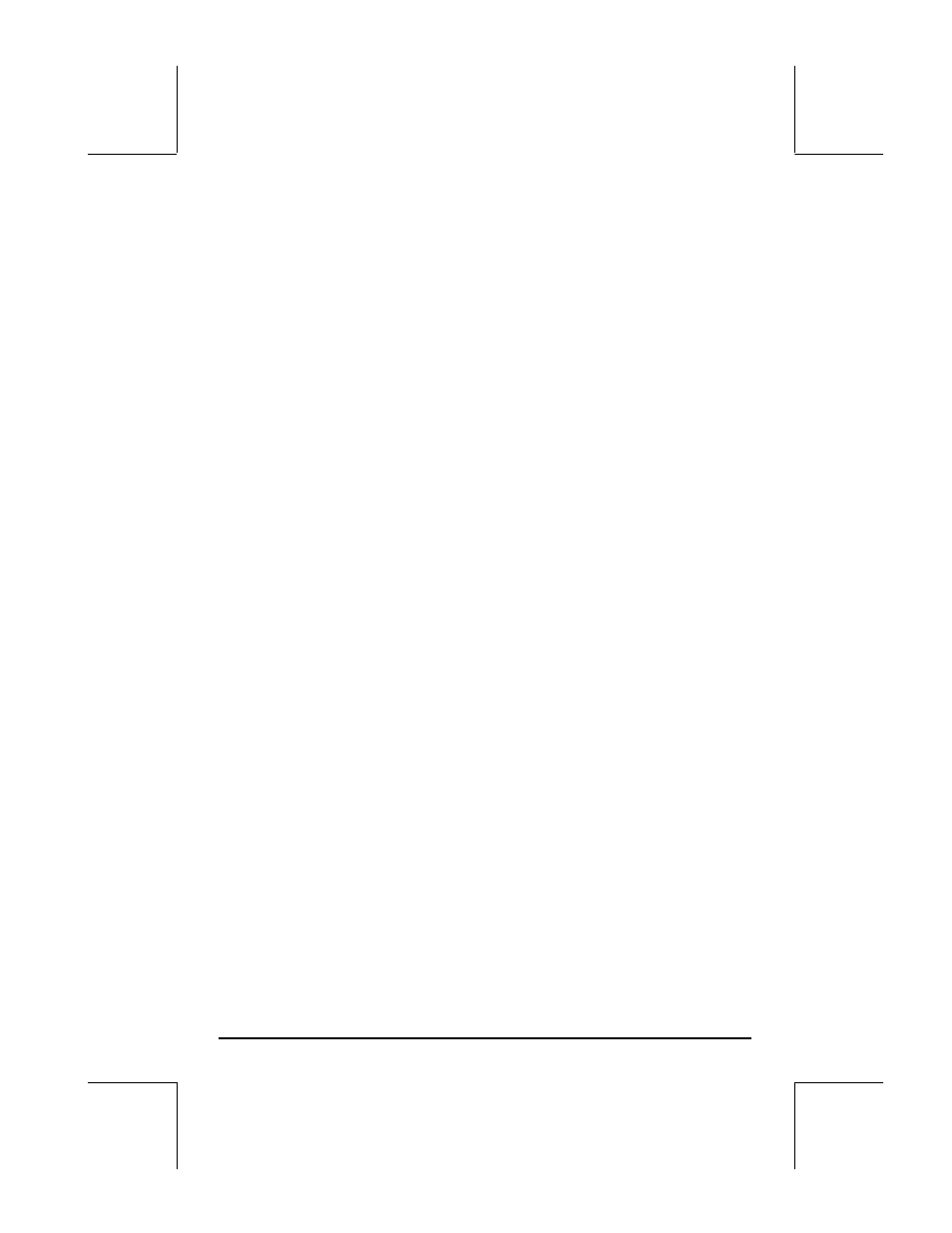
Page 18-24
The one-sided upper and lower 100(1-
α) % confidence limits for the
population mean
µ are, respectively, X+z
α
⋅σ/√n , and X−z
α
⋅σ/√n . Thus, a
lower, one-sided, confidence interval is defined as (-
∞ , X+z
α
⋅σ/√n), and an
upper, one-sided, confidence interval as (X
−z
α
⋅σ/√n,+∞). Notice that in
these last two intervals we use the value z
α
, rather than z
α/2
.
In general, the value z
k
in the standard normal distribution is defined as that
value of z whose probability of exceedence is k, i.e., Pr[Z>z
k
] = k, or Pr[Z k ] = 1 – k. The normal distribution was described in Chapter 17. Confidence intervals for the population mean when the Let X and S, respectively, be the mean and standard deviation of a random sample of size n, drawn from an infinite population that follows the normal σ. The 100⋅(1−α) % [i.e., 99%, 95%, 90%, etc.] central two-sided confidence interval for the population mean n-1, α /2 ⋅S /√n , X+ t n-1, α /2 ⋅S/√n ), where t n-1, α /2 is Student's t variate with ν = n-1 degrees of freedom and probability α/2 of exceedence. ⋅ (1-α) % confidence limits for the population mean µ are, respectively, X + t n-1, α /2 ⋅S/√n , and X− t n-1, α /2 ⋅S /√n. Small samples and large samples σ with S. Samples for which n>30 are typically referred to as large samples, otherwise they are small samples. Confidence interval for a proportion A discrete random variable X follows a Bernoulli distribution if X can take only
population variance is unknown
distribution with unknown standard deviation
µ, is (X− t
The one-sided upper and lower 100
The behavior of the Student’s t distribution is such that for n>30, the
distribution is indistinguishable from the standard normal distribution. Thus,
for samples larger than 30 elements when the population variance is unknown,
you can use the same confidence interval as when the population variance is
known, but replacing
two values, X = 0 (failure), and X = 1 (success). Let X ~ Bernoulli(p), where p