Bio-Rad Model 680 Microplate Reader Accessories User Manual
Page 93
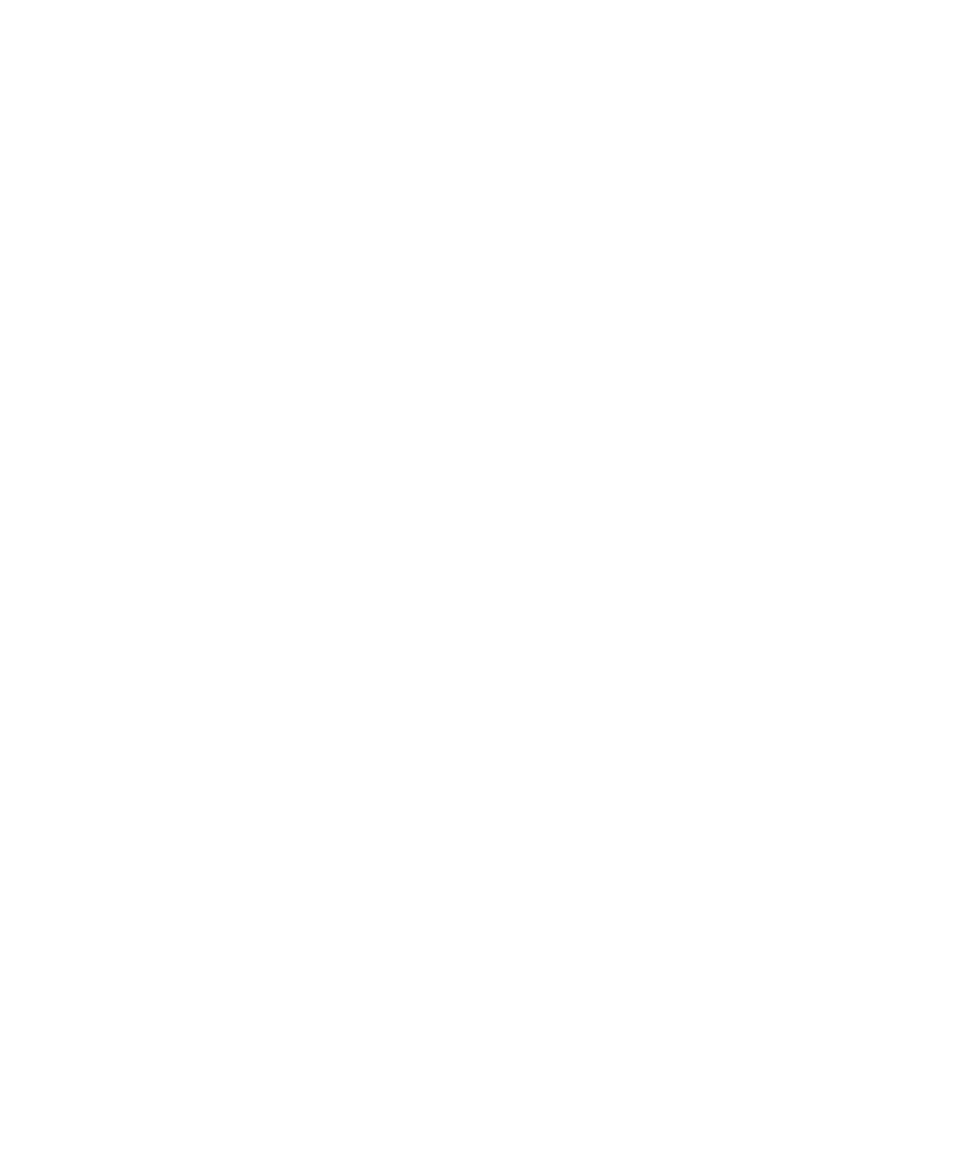
Chapter 6. Data Formatting and Reports
85
The polynomial regression models (Linear, Quadratic, and Cubic) and logistic
models are examples of global construction of a calibration function, while Cubic
Spline and Point to Point are examples of a local approach.
In general, when selecting a regression type for your data, you should start with the
simplest curve fitting routine (i.e., linear) and then examine all four Transformation
options (see below) for an acceptable curve fit. If a satisfactory fit is not obtained,
progressively increase the sophistication of the curve fitting until a good fit is
obtained.
Note that the equation for the selected regression method is displayed at the bottom
of the window. The concentration of unknowns is calculated by substituting the mean
absorbance values of the unknowns in the regression equation and solving for the
concentration.
For the Linear, Quadratic, and Cubic regression types, the correlation coefficient
associated with the equation gives an indication of how well the polynomial
regression model fits the data. The closer the correlation coefficient is to 1.000, the
better the fit of the regression.
Transformation
The selections under the Transformation pulldown menu change the scale of the
absorbance and concentration data between log and linear. Select from four different
combinations for calculating the curve: Linear(conc)-Linear(meas),
Linear(conc)-Log(meas), Log(conc)-Linear(meas), and Log(conc)-Log(meas).
Note that the logistic regression methods—Logistic 4PL, Logistic 5PL (Cook), and
Logistic 5PL (Rodbard)—are constrained to Linear-Linear transformation only. Also,
if you are plotting negative Kinetic velocities, then the velocity or maximum velocity
axis of the curve is constrained to linear unless the Negative Kinetics box is checked
(logarithms cannot be taken of negative values). See page 40 for details.