Google Understanding Visualization by Understanding Individual Users User Manual
Page 5
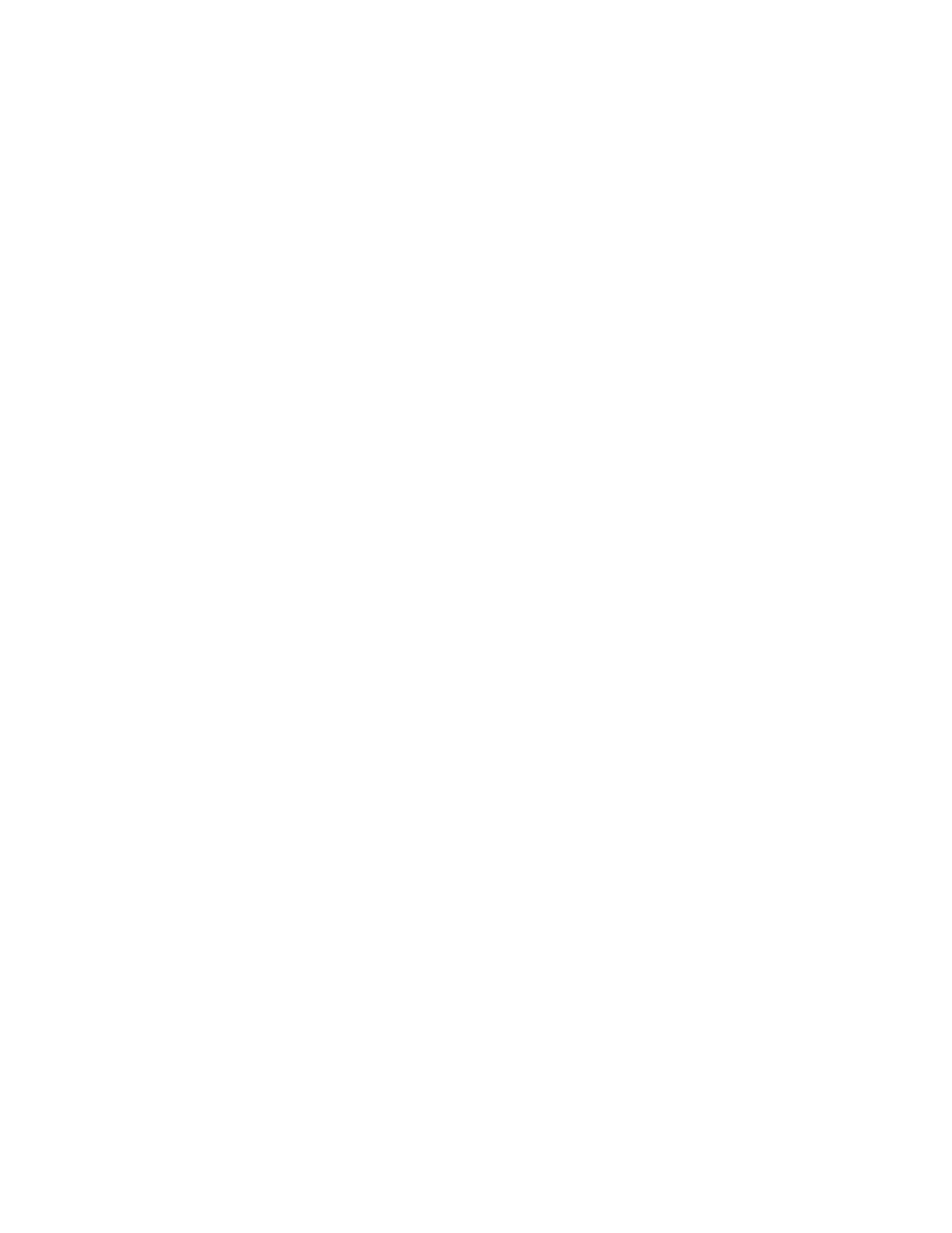
of usage patterns will allow us to extrapolate a user’s cognitive profile
and adapt the visual design accordingly.
While there exists a foundation of individual differences research
on which to build, in order to make progress toward adaptive visual-
izations, more work is needed to put this research in context. We must
understand what individual factors are important to visualization use
and develop methods for isolating design factors of a visualization.
Only with a clear understanding of both can we investigate why users
respond differently to different visualizations and apply that informa-
tion to designing for the user.
The challenges to designing for individuals are great, but the po-
tential benefits make this a challenge worth pursuing. At the indi-
vidual level, we each stand to benefit from systems that improve our
efficiency and accuracy. At the more global scale, many marginal-
ized and traditionally underserved user groups stand to benefit from
increased access to visualization systems tailored to them, rather than
those designed only for the average user. Finally, this research effort
will result in a much deeper understanding of how users make sense of
visual information. Visualizations are tools for thinking, and we can-
not understand visualization until we understand what people do with
those tools. Understanding that there is no one answer to that question
is an important step towards truly understanding visualization.
R
EFERENCES
[1] J. Bertin. Semiology of Graphics. University of Wisconsin Press, 1967.
[2] S. K. Card and J. Mackinlay. The structure of the information visual-
ization design space. In IEEE Symposium on Information Visualization,
pages 92–99, 1997.
[3] C. Chen and M. Czerwinski. Spatial ability and visual navigation: an
empirical study. New Review of Hypermedia and Multimedia, 3(1):67–
89, 1997.
[4] C. A. Cohen and M. Hegarty. Individual differences in use of external
visualisations to perform an internal visualisation task. Applied Cognitive
Psychology
, 21:701–711, 2007.
[5] C. Conati and H. Maclaren. Exploring the role of individual differences
in information visualization. In Advanced Visual Interfaces. ACM Press,
2008.
[6] T. Green and B. Fisher. Towards the personal equation of interaction: The
impact of personality factors on visual analytics interface interaction. In
Visual Analytics Science and Technology (VAST), 2010 IEEE Symposium
on
, pages 203–210. IEEE, 2010.
[7] T. Green, D. Jeong, and B. Fisher. Using personality factors to predict
interface learning performance. In hicss, pages 1–10. IEEE Computer
Society, 1899.
[8] I. A. Khan, W.-P. Brinkman, N. Fine, and R. M. Hierons. Measuring per-
sonality from keyboard and mouse use. In Proceedings of the European
Conference on Cognitive Ergonomics
, 2008.
[9] P. Langley. User modeling in adaptive interfaces. In Proceedings of User
Modeling
, pages 357–370, 1999.
[10] S. Pinker. A theory of graph comprehension. In R. O. Freedle, editor,
Artificial Intelligence and the Future of Testing
, pages 73–126. Lawrence
Erlbaum Associates, 1990.
[11] K. E. Pocius. Personality factors in human-computer interaction: A re-
view of the literature. Computers in Human Behavior, 7:103–135, 1991.
[12] B. W. Roberts and W. F. DelVecchio. The rank-order consistency of per-
sonality traits from childhood to old age: A quantitative review of longi-
tudinal studies. Psychological Bulletin, 126(1):3–25, 2000.
[13] J. B. Rotter. Generalized expectancies for internal versus external control
of reinforcement. Psychological Monographs, 80(609), 1966.
[14] B. Saati, M. Salem, and W.-P. Brinkman. Towards customized user inter-
face skins: investigating user personality and skin colour. In HCI 2005,
pages 89–93, 2005.
[15] B. Shneiderman. The eyes have it: A task by data type taxonomy for
information visualizations. In IEEE Symposium on Visual Languages,
pages 336–343, 1996.
[16] M. Tory and T. M¨oller. Rethinking visualization: A high-level taxonomy.
In IEEE Symposium on Information Visualization, pages 151–158, 2004.
[17] M. C. Velez, D. Silver, and M. Tremaine. Understanding visualization
through spatial ability differences. In IEEE Visualization, pages 511–
518, 2005.
[18] J. S. Yi. Implications of individual differences on evaluating information
visualization techniques. In Proceedings of the BELIV Workshop, 2010.
[19] C. Ziemkiewicz, R. J. Crouser, A. R. Yauilla, S. L. Su, W. Ribarsky, and
R. Chang. How locus of control influences compatibility with visualiza-
tion style. In IEEE Visual Analytics Science and Technology, 2011.
[20] C. Ziemkiewicz and R. Kosara. Preconceptions and individual differ-
ences in understanding visual metaphors. Computer Graphics Forum,
28(3):911–918, 2009. Proceedings EuroVis.