1 calibration development, 1 simple linear regression, 1 overview – Metrohm Vision – Theory User Manual
Page 7: 2 correlation, 3 sensitivity, Calibration development, Simple linear regression, Overview, Correlation, Sensitivity
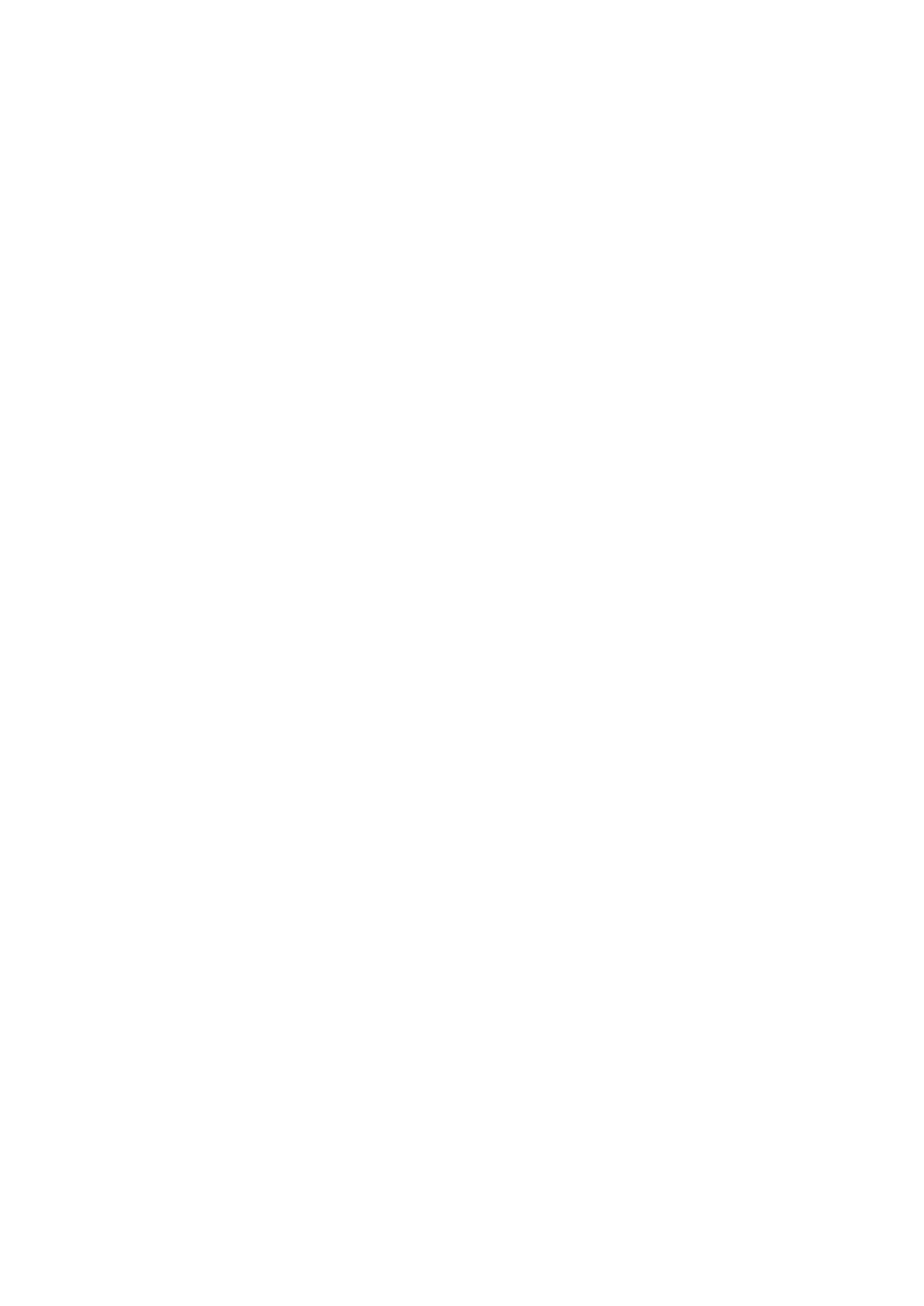
▪▪▪▪▪▪▪
5
1
Calibration Development
1.1
Simple Linear Regression
1.1.1
Overview
The simplest method of calibration is based on a single independent variable (wavelength). Vision
uses the Inverted Least Squares (ILS) method. The assumption is that constituent values c (usually
concentrations) are a linear function of the absorbance A at some wave-length i:
i
A
K
K
c
⋅
+
=
)
1
(
)
0
(
(This expression contrasts the Lambert-Beer Law, which gives absorbance as a function of
concentration, and hence is referred to as “inverted.”)
ILS is presented graphically by a least squares fit of a straight line to data points in plot of
concentration versus absorbance. The least squares approach fits the line so as to minimize the sum
of squares of deviations between data points and the calibration line; it yields two calibration
constants: intercept K(0) and slope K(1). Those deviations are taken along the concentration axis, and
so are called concentration residuals.
1.1.2
Correlation
The wavelength at which the calibration is performed is usually selected because of high correlation
between concentration and absorbance: correlation is a measure of how well the data at a certain
wavelength is represented by the linear equation. Correlation can range between -1 and 1, with
values at either extreme indicating that the linear model does indeed describe the data set (there is a
good fit between the concentration-absorbance data and the linear equation).
Often a high absolute value for correlation at some wavelength confirms what is visually evident
when sample spectra in a calibration set are overlaid. Such visual evaluation of spectral data is
important to maximize confidence that the model developed is indeed appropriate. For, though
statistics are important and useful tools, they should be used to help make optimal choices rather
than blind choices.
A value for correlation falling in the middle of the range (correlation is close to zero) indicates lack of
relationship between constituent and spectral data at this wavelength. In other words, the linear
model does not describe the data set. Vision contains tools to select the calibration wavelength that
gives the best correlation.
1.1.3
Sensitivity
Another important property of a calibration is sensitivity. Sensitivity is the absolute value of the
slope term in the calibration equation. If the sensitivity is very high, even small changes in
absorbance, e.g. those caused by noise, will register as a large change in predicted concentration. On
the other hand, low sensitivity indicates a robust calibration that gives stable and reliable predictions.
Of course, low sensitivity is useful only when correlation is very high, for low sensitivity with low
correlation would have little predictive value. Superimposed plots of correlation and sensitivity values
across the full spectral range are helpful in visualizing which wavelength(s) is (are) best for calibration.
Thus, that wavelength is best which has a correlation close to 1 or -1, as well as low sensitivity.