Metrohm Vision – Theory User Manual
Page 16
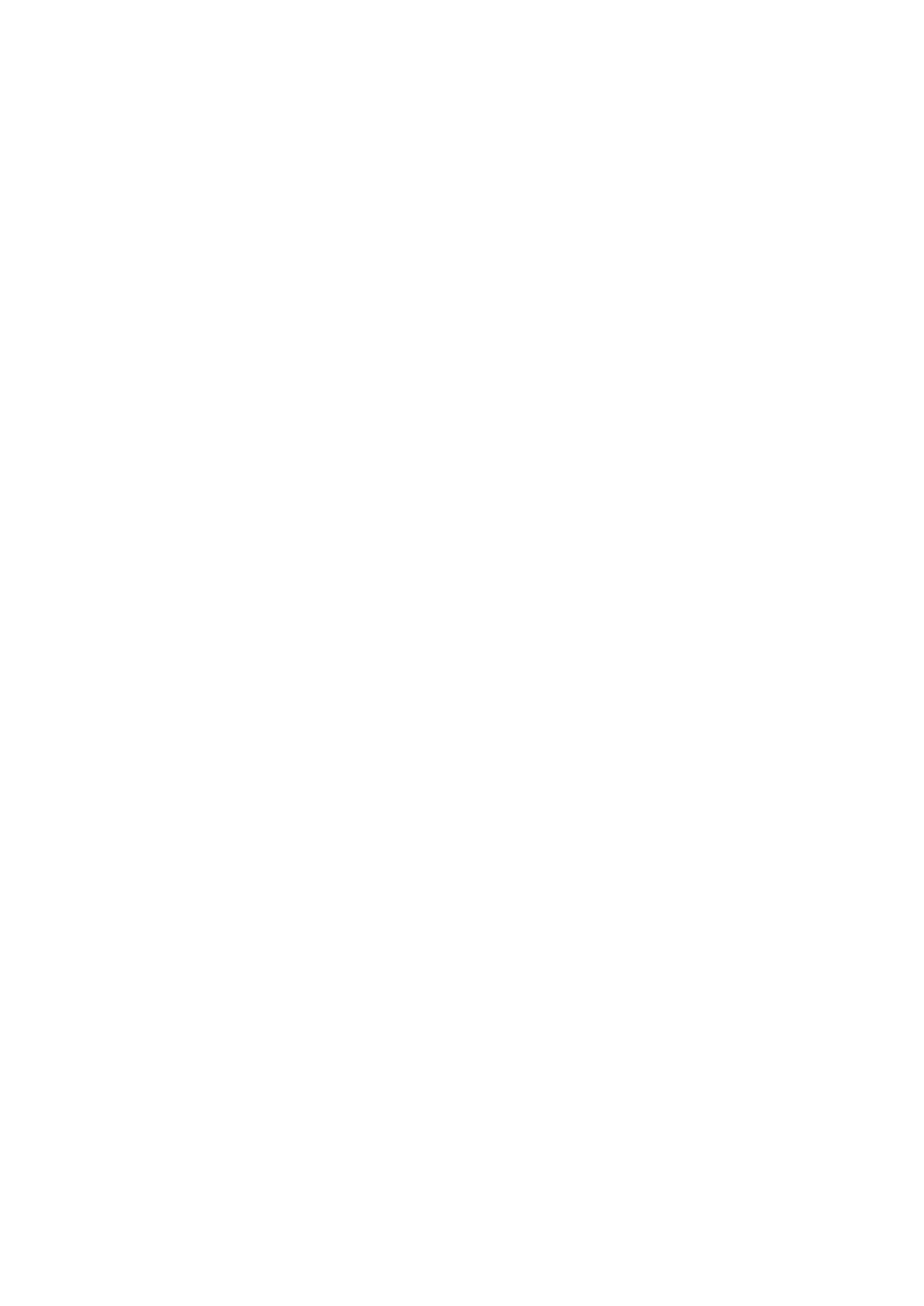
14
▪▪▪▪▪▪▪
linear regression is performed on absorbance values of the sample spectrum versus those at
corresponding wavelengths in the mean spectrum. This operation yields a linear equation with a
defined intercept and slope. Next, the value of the intercept is subtracted from every data point in
the spectrum. Finally, each absorbance value in the resulting spectrum is divided by the value for
slope. Using the mean spectrum, this same set of operations is performed on every spectrum in the
data set.
Pretreatments like derivatives, SNV, Detrend, and Savitsky-Golay are applied to individual spectra
without any preconception of what the resulting spectrum should look like. By contrast, the MSC
method calculates a mean spectrum under the assumption that spectra in the data set are distributed
normally. Thus, the mean spectrum is the most probable spectrum, i.e. it is the highest probability
representation of all spectra in the data set.
MSC effectively coerces individual spectra to behave like the mean spectrum as much as possible.
Success of the method strongly depends on the calculated mean spectrum closely resembling the
true mean spectrum, which depends in turn on a large sample set. Quantitative method development
usually requires such data sets to completely span the full range of chemical and spectral variation
encountered when the method is actually implemented.
Sample sets for qualitative models development also may be large – indeed, they may include many
times more samples than quantitative models – because they often include dozens of hundreds of
products. However, a given product may contain only a few samples. Consequently, MSC may not be
as suitable for qualitative analysis as it is for those quantitative applications where elimination of
pathlength and slope variation in a single pretreatment is important.