2 maximum distance in wavelength space, 1 outlier detection, 2 redundant sample selection – Metrohm Vision – Theory User Manual
Page 21: 3 random selection, Maximum distance in wavelength space, Outlier detection, Redundant sample selection, Random selection
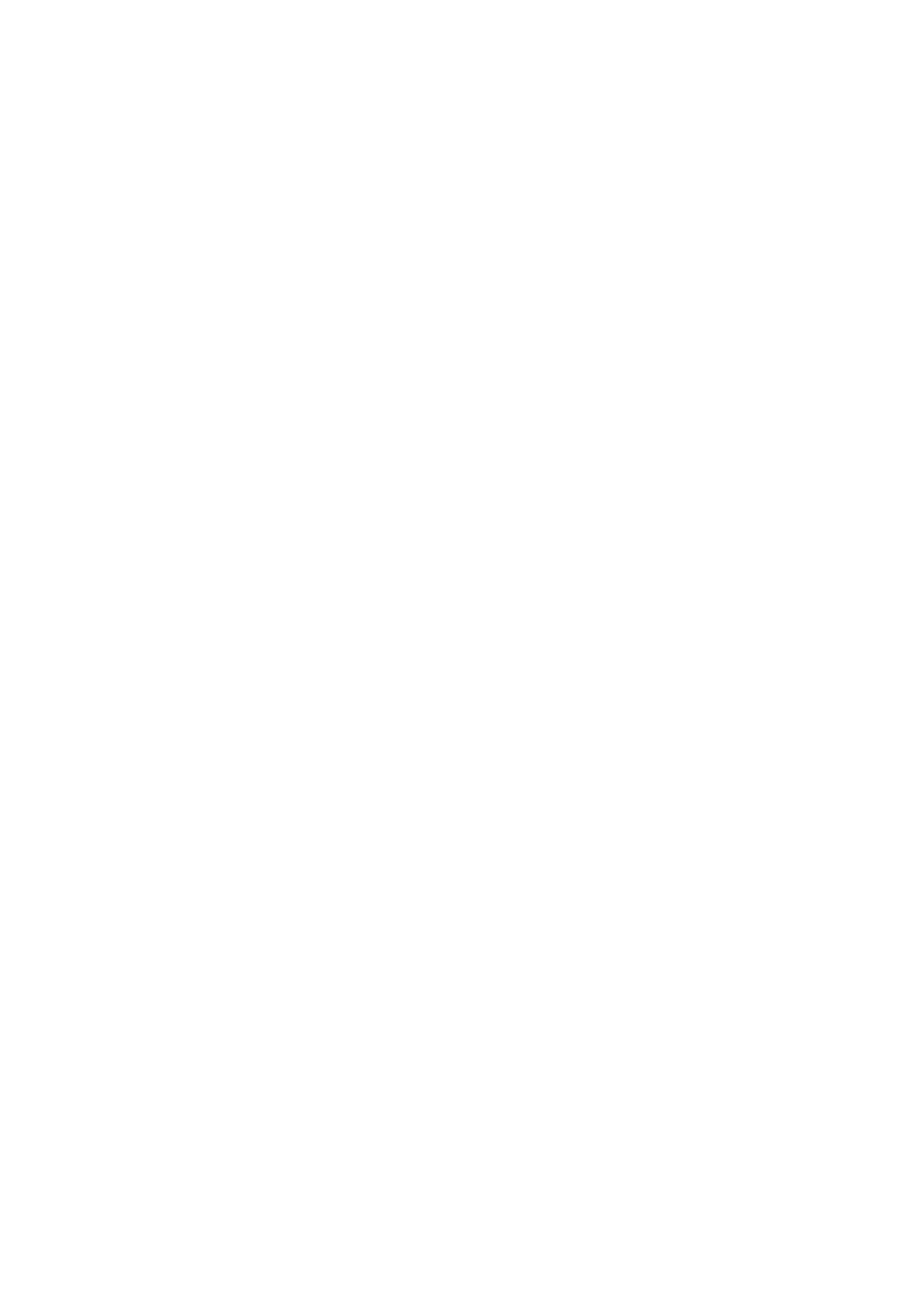
▪▪▪▪▪▪▪
19
estimates the what threshold is required to obtain the desired distribution of samples between
training and acceptance sets.
Before the sample selection is performed, the data set may include outliers. Because the presence of
outliers distorts the Principal Component model, optimally the outliers should be removed from the
set as soon as they are detected, and the model recalculated before redundant samples are found.
This can be done by selection the Reset Product Mean option during Sample Selection.
If this is the case, Vision will display on all plots the results of the second method, without outliers.
However, if the outliers are found, they are saved in the Rejection Set.
If you want to see the outliers on plots, do not select the Reset Product Mean option. However, be
aware that redundant samples search is done within the model that may include outliers.
4.2
Maximum Distance in Wavelength Space
4.2.1
Outlier Detection
In this method outliers are detected by calculating maximum distance of all product spectra from the
product mean spectrum. The standard deviation envelope is defined using all product spectra.
4.2.2
Redundant Sample Selection
Redundant samples are detected based on Euclidean distances in wavelength space (calculated on
spectra). After removal of outlier samples, remaining samples undergo redundant sample selection.
If the distance threshold method is used to select redundant samples, Vision randomly picks a
spectrum and calculates distances from this spectrum to all other spectra. This spectrum is placed in
the training (or calibration) set, and all spectra with distances smaller than the threshold are placed in
the acceptance (validation) set. The process continues until all spectra are distributed between
appropriate sets.
Because the calculated distances are not scaled, threshold values depend on the product spectra.
Therefore, to optimize sample selection for a given product, several runs may be required. For this
reason, By Number of Samples is the preferred option for sample selection. In this case Vision
estimates the what threshold is required to obtain the desired distribution of samples between
training and acceptance sets.
Before the sample selection is performed, the data set may include outliers. Because the presence of
outliers distorts the Principal Component model, optimally the outliers should be removed from the
set as soon as they are detected, and the model recalculated before redundant samples are found.
This can be done by selection the Reset Product Mean option during Sample Selection.
If this is the case, Vision will display on all plots the results of the second method, without outliers.
However, if the outliers are found, they are saved in the Rejection Set.
If you want to see the outliers on plots, do not select the Reset Product Mean option. However, be
aware that redundant samples search is done within the model that may include outliers.
4.3
Random Selection
When this method of sample selection is chosen, Vision randomly splits all the product spectra into
training and acceptance sets. No rejection (outlier) set is created when this method is applied.